Blog
Game Theory vs. Agent-Based Modeling: Which is Better for Social Simulations?
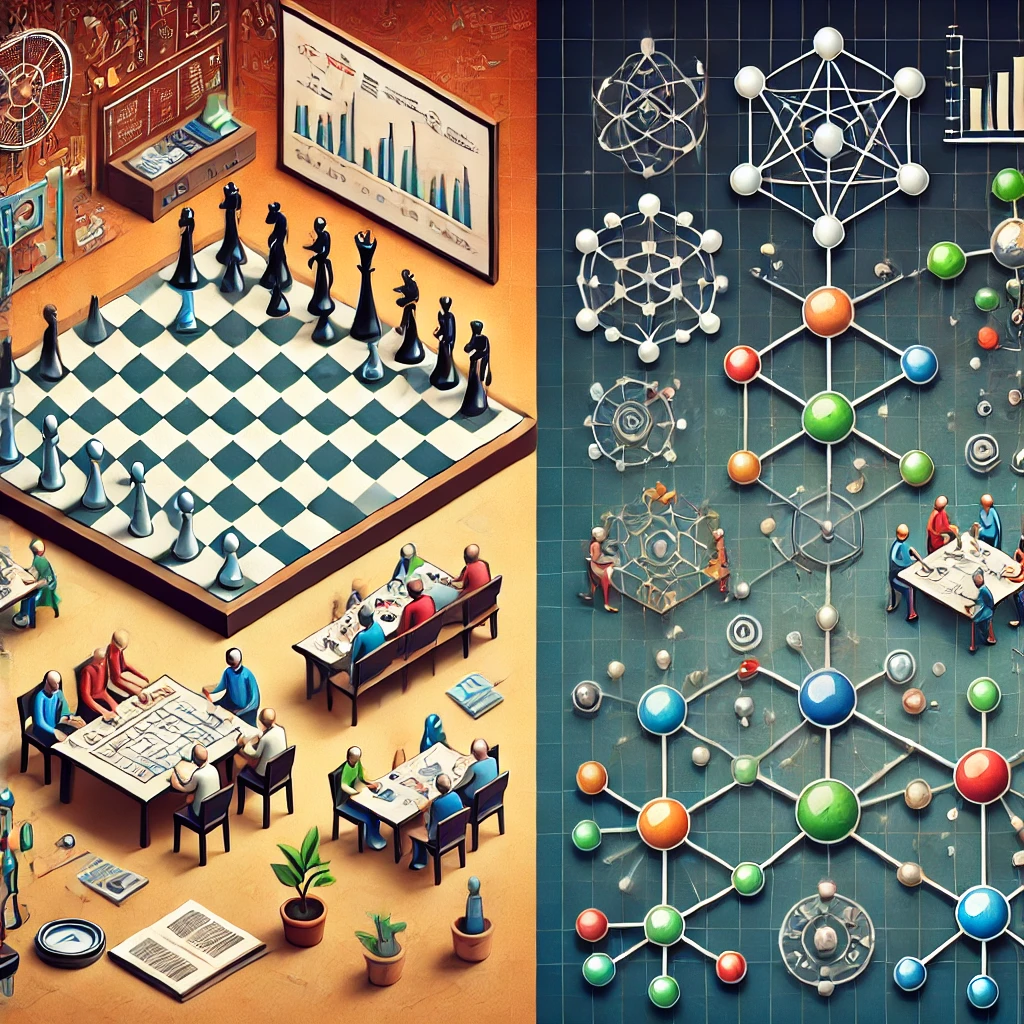
Social simulations have become indispensable tools for understanding complex human behaviors, predicting societal trends, and designing effective policies. Two prominent approaches dominate the field: game theory and agent-based modeling (ABM). While both have their strengths, the question remains: which approach is better suited for social simulations? The answer is not straightforward, as it largely depends on the specific goals, context, and complexity of the simulation. Let’s delve into the core principles, advantages, limitations, and applications of both approaches to provide clarity.
Understanding Game Theory
Game theory is a mathematical framework used to analyze decision-making in strategic situations where the outcomes depend on the actions of multiple agents. Its foundation lies in concepts such as Nash equilibrium, payoff matrices, and utility maximization.
Advantages of Game Theory
- Simplicity and Clarity: Game theory offers clear, mathematical structures that are easy to interpret. Models like prisoner’s dilemma or public goods games provide concise insights into strategic interactions.
- Analytical Rigor: With its strong mathematical foundation, game theory ensures precise and logical reasoning, making it ideal for understanding competitive and cooperative behaviors.
- Wide Applicability: Game theory has been successfully applied in economics, political science, and evolutionary biology to study phenomena like market competition, voting systems, and resource allocation.
Limitations of Game Theory
- Static Assumptions: Traditional game theory often assumes rational agents with complete information, which oversimplifies real-world human behaviors.
- Lack of Dynamism: It struggles to capture the evolving and adaptive nature of social systems over time.
- Limited Realism: The rigid structure of game theory can exclude nuanced behaviors like emotions, cultural norms, and bounded rationality.
Understanding Agent-Based Modeling (ABM)
Agent-based modeling is a computational approach where autonomous agents interact within a simulated environment. Each agent follows a set of rules and adapts its behavior based on interactions with other agents and the environment.
Advantages of ABM
- High Flexibility: ABM allows for the incorporation of diverse and heterogeneous agents, making it highly adaptable to complex social systems.
- Dynamic and Emergent Behaviors: It captures how individual interactions lead to emergent macro-level patterns, providing insights into phenomena like crowd behavior, diffusion of innovation, or social networks.
- Behavioral Realism: ABM can model agents with bounded rationality, emotions, and even irrational behaviors, closely mimicking real-world scenarios.
Limitations of ABM
- Computational Intensity: Running large-scale simulations can require significant computational resources and time.
- Complexity in Validation: Unlike game theory, validating ABM models against empirical data can be challenging due to their complexity.
- Subjectivity in Rules: Defining the rules and parameters for agents can introduce bias and reduce objectivity.
Comparing Game Theory and ABM for Social Simulations
1. Nature of the Problem
- Game theory is well-suited for situations with strategic decision-making and clear, predictable payoffs (e.g., negotiations, market competition).
- ABM excels in modeling complex, adaptive systems where individual behaviors and interactions drive emergent outcomes (e.g., urban development, social movements).
2. Realism vs. Abstraction
- Game theory provides a highly abstract representation, focusing on essential strategic elements.
- ABM emphasizes realism by simulating detailed individual behaviors and interactions.
3. Computational Demands
- Game theory’s analytical nature requires minimal computational power and is faster to implement.
- ABM, being computationally intensive, is more resource-demanding but offers richer insights into dynamic processes.
4. Scalability
- Game theory can struggle with scalability as the number of agents and strategies increases.
- ABM handles scalability better, as it thrives on simulating large populations and their interactions.
When to Choose Game Theory
Game theory is the better choice when:
- The problem involves strategic decision-making among a small number of rational agents.
- The primary goal is to derive clear and generalizable insights.
- Time and computational resources are limited.
Example Application: In political science, game theory has been used to model voting strategies, where candidates and voters behave strategically to maximize their outcomes.
When to Choose ABM
ABM is more suitable when:
- The simulation involves complex, adaptive systems with heterogeneous agents.
- Emergent behaviors and dynamic interactions are of interest.
- Behavioral realism is critical to the study.
Example Application: In sociology, ABM has been used to study the spread of cultural norms in diverse populations, capturing the influence of peer interactions and local contexts.
The Best of Both Worlds: Combining Game Theory and ABM
Rather than choosing one over the other, combining game theory and ABM can leverage the strengths of both approaches. Game theory can provide the strategic foundation for agent behaviors, while ABM can simulate how these strategies evolve in dynamic and complex environments.
Example: In economics, a hybrid model might use game theory to define the strategic choices of firms in a competitive market, while ABM simulates consumer behaviors and market dynamics over time.
Conclusion: Context Matters
The debate over whether game theory or agent-based modeling is better for social simulations does not have a one-size-fits-all answer. The choice depends on the specific objectives, context, and constraints of the simulation. While game theory offers clarity and simplicity, ABM provides depth and realism. Recognizing the strengths and limitations of each approach allows researchers and policymakers to make informed decisions that best address their goals.
-
Software3 years ago
Five Productivity Tools That You Will Need in 2022 and Why
-
News2 years ago
An Open Source 2.5-Inch IDE Solid-State Drive is a Necessity for Your Old ThinkPad
-
News6 years ago
Battlefield 5 Chapter Reward Catch-ups are Now Accessible
-
Apple3 years ago
How to Find People and Faces in the Photos App on iPhone or iPad